
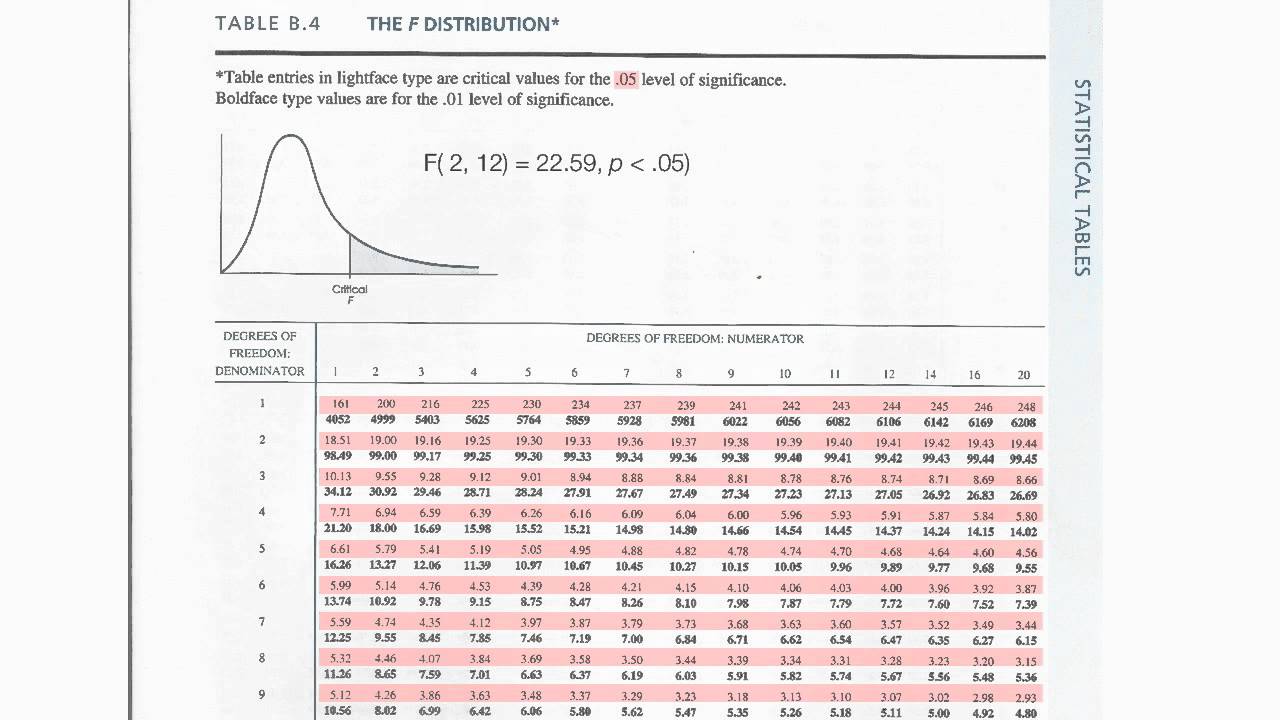
To look at the relationship among the variables, we can calculate the correlation matrix using the correlation function cor().īased on the correlation matrix, the correlation between college GPA and high school GPA is about 0.545, which is larger than that (0.523) between college GPA and SAT, in turn larger than that (0.35) between college GPA and quality of recommendation letters. The calculation and interpretation of the sample product moment correlation. Those numbers can be obtained using the summary() function. For each variable, we calculate 6 numbers: minimum, 1st quartile, median, mean, 3rd quartile, and maximum. Next, we can calculate some summary statistics to explore our data further. The relationship is close to linear and the relationship seems to be stronger for high school GPA and SAT than for the quality of recommendation letters. From the plots, we can roughly see all three predictors are positively related to the college GPA. The scatterplots between college GPA and the three potential predictors are given below.
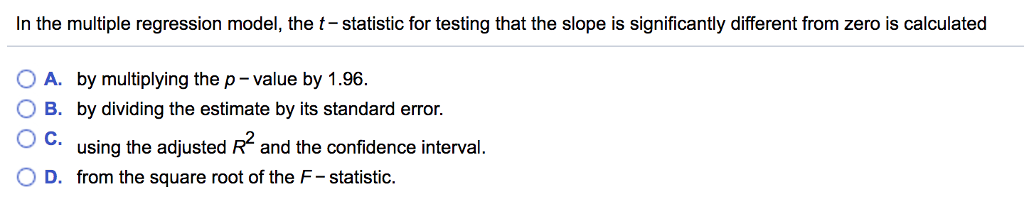
A scatterplot can tell us the form of relationship, e.g., linear, nonlinear, or no relationship, the direction of relationship, e.g., positive or negative, and the strength of relationship, e.g., strong, moderate, or weak. > usedata('gpa')īefore fitting a regression model, we should check the relationship between college GPA and each predictor through a scatterplot. As shown below, the sample size is 100 and there are 4 variables: college GPA (c.gpa), high school GPA (h.gpa), SAT, and quality of recommendation letters (recommd). In this example, we use a set of simulated data (generated by us). For the sake of this example, let's measure college success using college GPA. Should admissions officers pay most attention to more easily quantifiable measures such as high school GPA and SAT scores? Or should they give more weight to more subjective measures such as the quality of letters of recommendation? What are the pros and cons of the approaches? Of course, how we define college success is also an open question. Why might we want to do this? There's an ongoing debate in college and university admission offices (and in the courts) regarding what factors should be considered important in deciding which applicants to admit. An exampleĪs an example, suppose that we wanted to predict student success in college. Intuitively, this test determine whether the variance explained by the first \(p\) predictors above and beyond the $k-p$ predictors is significance or not. Multiple regression modelĪ general multiple linear regression model at the population level can be written as Psychologists may want to determine which personality dimensions best predicts social adjustment. For example, educational researchers might want to learn what the best predictors of success in college are. Multiple regression allows a researcher to ask (and hopefully answer) the general question "what is the best predictor of. In the social and natural sciences, multiple regression analysis is very widely used in research. Multiple regression analysis often focuses on understanding (1) how much variance in a DV a set of IVs explain and (2) the relative predictive importance of IVs in predicting a DV. The general purpose of multiple regression (the term was first used by Pearson, 1908), as a generalization of simple linear regression, is to learn about how several independent variables or predictors (IVs) together predict a dependent variable (DV).
